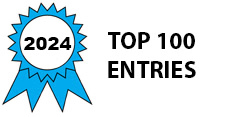
In the rapidly evolving aviation landscape, technological advancements are crucial in enhancing operational efficiency, safety, and profitability. Kquika, a pioneer in artificial intelligence (AI) and machine learning (ML) solutions, is at the forefront of this transformation. By leveraging sophisticated algorithms and innovative technologies, Kquika is revolutionizing the aerospace/aviation market, setting new standards for predictive maintenance, flight optimization, and air traffic management with the ability to predict flight delays and disruptions caused by weather or mechanical failures with 95% accuracy.
Kquika’s two key technologies are a Predictive Maintenance Platform and a Disruption and Recovery Platform:
The Predictive Maintenance Platform harnesses extensive use and repair data from various commercial aircraft models. It predicts the need for maintenance, allowing airlines to address potential issues before they escalate into costly failures. This proactive approach reduces maintenance costs and minimizes the risk of operational disruptions, ensuring passenger and crew safety.
The Disruption and Recovery Platform integrates comprehensive flight data—including weather conditions, planned and actual flight paths, and air traffic control information. Powered by sophisticated machine learning algorithms, it anticipates and manages potential delays. When disruptions are detected, the system promptly notifies customers and facilitates swift rebooking options, eliminating the need for direct human interaction. This efficiency reduces staffing costs and enhances passenger experience by reducing wait times and uncertainty.
Advanced Machine Learning: Kquika’s Impact
Machine learning, a subset of AI, involves training algorithms on vast datasets to recognize patterns, make decisions, and predict future outcomes accurately. Kquika's approach to machine learning is multifaceted, addressing various aspects of aviation to create a cohesive, intelligent ecosystem.
1. Predictive Maintenance: Reducing Downtime and Costs
Predictive maintenance is one of the most critical applications of machine learning in aviation. Traditionally, aircraft maintenance follows a schedule based on flight hours or cycles, which can lead to unnecessary downtime and inflated costs. Kquika's ML models analyze real-time data from aircraft sensors, historical maintenance records, and environmental factors to predict when/where failures might occur. By implementing predictive maintenance, Kquika helps airlines:
- Reduce Unscheduled Downtime
- Optimize Maintenance Schedules
- Lower Maintenance Costs
2. Flight Optimization: Enhancing Efficiency
Fuel consumption and route efficiency are paramount concerns in aviation, directly impacting operational costs and environmental footprint. Kquika's algorithms optimize flight paths by analyzing many variables, including weather conditions, air traffic, and aircraft performance.
Key benefits of Kquika's flight optimization solutions:
- Fuel Efficiency: Identifying the most fuel-efficient routes/altitudes
- Time Savings: Optimized flight paths
- Dynamic Adjustments: The ability to adapt to real-time conditions
3. Air Traffic Management: Improving Safety and Capacity
Air traffic management (ATM) is another critical area where Kquika's technology makes a difference. With increasing air traffic, managing the flow of aircraft safely and efficiently is a significant challenge. Ultimately supporting air traffic controllers and minimizing human error.
Benefits of Kquika's ATM solutions include:
- Enhanced Safety: Early warnings of potential conflicts and deviations
- Increased Capacity: Optimized flight sequencing and spacing
- Improved Efficiency: Real-time analysis and decision-making
-
Awards
-
2024 Top 100 Entries
Voting
-
ABOUT THE ENTRANT
- Name:Victor Oribamise
- Type of entry:teamTeam members: