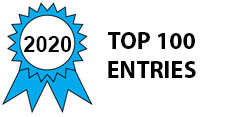
Chemical manufacturing is responsible for close to 30% of the global energy demand, and innovative solutions are needed to reduce the impact of CO2 emissions released from energy-intensive chemical processes. Electrochemical processes have emerged as a promising approach that replaces the use of heat and hazardous reagents from traditional thermal transformations with benign electrons that can more easily be derived from renewable sources. Organic electrosynthesis is a growing field that improves competitiveness, efficiency, and sustainability of chemical manufacturing, as it can lower resource and energy consumption, reduce the number of reaction steps, lower waste production, and, in many cases, open access to untapped reaction pathways to new substances addressing unmet needs.
In spite of these advantages, there are several challenges that prevent the wide implementation of these more efficient electrochemical manufacturing routes. First, developing and optimizing electrochemical reactions requires extensive theoretical and practical expertise, or, at the very least, considerable resources to gain empirical understanding. Second, since electrochemical manufacturing is affected by many different process variables needing small adjustments (e.g. reactant concentrations, electrode potential, temperature, pressure, flow rates), traditional process discovery and optimization normally requires hundreds-to-thousands of experiments that demand expensive human and material resources. Third, available statistical modeling approaches to predict reaction performance are very computationally expensive or do not correctly capture the complex physical behavior observed in electrochemical systems.
In order to propel the adoption of electrosynthesis and revolutionize chemical manufacturing, Sunthetics offers equipment and tools to lower the barrier to implementation of electrochemical processes. We have developed versatile, easy-to-use, large-scale (kg/day scale) reactors that are designed to enhance efficiency in electrosynthesis and we couple them with machine-learning (ML) tools that accelerate process optimization. The use of the ML platform allows the user to collect small experimental datasets (limiting costs and time committed to process development) and use them to predict reaction performance accurately for all combinations of operating conditions, accelerating the identification of optimal points that maximize efficiency. This technology was tested in the production of nylon intermediates, and it reduced raw material and energy use by 30%, with a reduction of 30% in emissions. These advantages further led to a 20% reduction in manufacturing costs.
We have raised $400K in non-dilutive grants and competitions to scale up our technology and we have already grown 100x in size (1 to 100 cm2 active area) without any losses in efficiency. We are now partnering with pharmaceutical companies to pilot our technology in their laboratories, advancing the development of more sustainable electrochemical production routes for pharmaceutical molecules. Their molecule development teams are interested in implementing electrochemical processes, but lack the equipment and expertise to rapidly implement new chemical manufacturing routes, opening an entry to market for our technology. Given that this is a versatile approach, it can be used in a multitude of chemical reactions. We plan on becoming a platform that will influence the chemical industry as a whole, making it sustainable, one reaction at a time.
Video
-
Awards
-
2020 Top 100 Entries
Voting
-
ABOUT THE ENTRANT
- Name:Daniela Blanco
- Type of entry:teamTeam members:Daniela Blanco - CTO, Technology Developer
Myriam Sbeiti - CEO - Profession:
- Daniela is inspired by:Artificial Intelligence is the key to advance the implementation of sustainable chemical processes and open opportunities for a new generation of sustainable chemical manufacturing.
- Software used for this entry:Matlab, Python
- Patent status:none