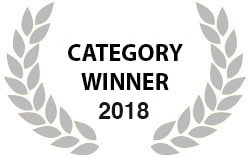
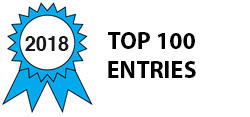
The Implantable Myoelectric Sensor (IMES) system developed by the Alfred Mann Foundation is an implantable sensor technology platform capable of transmitting localized myoelectric signals simultaneously from multiple muscle sources, and is intended to be integrated into prosthetic systems to control electromechanical prosthetic devices. The IMES implants detect myoelectric signals generated by residual muscles of an amputated limb, which are still under control of the brain. These signals are wirelessly transmitted to an external processor that controls the movement of a prosthetic device. Control can be intuitive by assigning the muscle’s signal to a prosthetic function that correlates with the hand function that muscle provided prior to its amputation.
Currently available myoelectric prostheses use these same signals, but they are detected from the surface of the skin, which results in poor specificity, repeatability and reliability due to crosstalk, electrode liftoff and sweat. Current systems can typically only pick up one or two signals. The patient has to use these same signals to control a variety of different movements, which can only be activated one at a time. The user has to signal a mode change to switch between the different functions. This kind of sequential control is slow and non-intuitive. These limitations are addressed by the IMES system using permanently implanted sensors to detect signals from within the muscles.
The IMES are tiny implants that consist of custom electronics housed in hermetically sealed, biocompatible ceramic capsules with metal endcaps. Each one is only 16 mm long and 2.5 mm in diameter. IMES are inductively powered through a magnetic field produced by a coil laminated inside the wall of a prosthetic socket frame. Bidirectional telemetry is achieved through modulation of this field. Each implant amplifies, filters and transmits the detected signal to a Telemetry Controller, or TC. In the first generation product, which was clinically demonstrated in patients with transradial, transhumeral, transtibial and transfemoral amputation, the TC was incorporated into a belt pack connected to the coil by a cable, but improvements have since been made that allow for integration of the TC in the prosthetic frame housing. The TC separates out the samples from each of the implants, reconstructs them into an analog envelope, and then routs each one to a pre-assigned motor of the prosthesis to effect a particular movement. The different movements can all occur simultaneously. In the second generation product, raw digital samples of signals acquired from up to 16 devices can be fed to a controller that uses machine learning to determine which muscles have contracted and either activate different hand grasps or perform individual finger control of a robotic hand.
At present, the limitation of advanced myoelectric prostheses is not their ability to reproduce near-normal human movements, but rather the lack of an effective means to control the multitude of actions they are capable of. IMES implanted into residual musculature address the core issue of how to extract multi-channel biological signals that are stable and reliable over long periods of time.
Video
-
Awards
-
2018 Medical Category Winner
-
2018 Top 100 Entries
Voting
-
ABOUT THE ENTRANT
- Name:Patrick Nercessian
- Type of entry:teamTeam members:Alanie Atyabi, Brian Dearden, Dave Brown, Dave Melbye, Dianna Han, Ed Hillery, Emil Istoc, Greg Golamirians, Harshit Suri, Jaime Gutierrez, Joseph Calderon, Justin Loo, Mike Perrin, Patrick Nercessian, Sam Bowman, Sam Yang, Taunyia Woolfolk, Valma Klein, Yesenia Acevedo
- Profession:
- Number of times previously entering contest:never
- Patrick is inspired by:The knowledge that the designs I contribute to will lead to products that have a significant impact on the quality of life of individuals with medical needs.
- Software used for this entry:COMSOL, Cadence EDA tools, SolidWorks, Matlab, Python, Atollic, Visual Studio
- Patent status:patented